
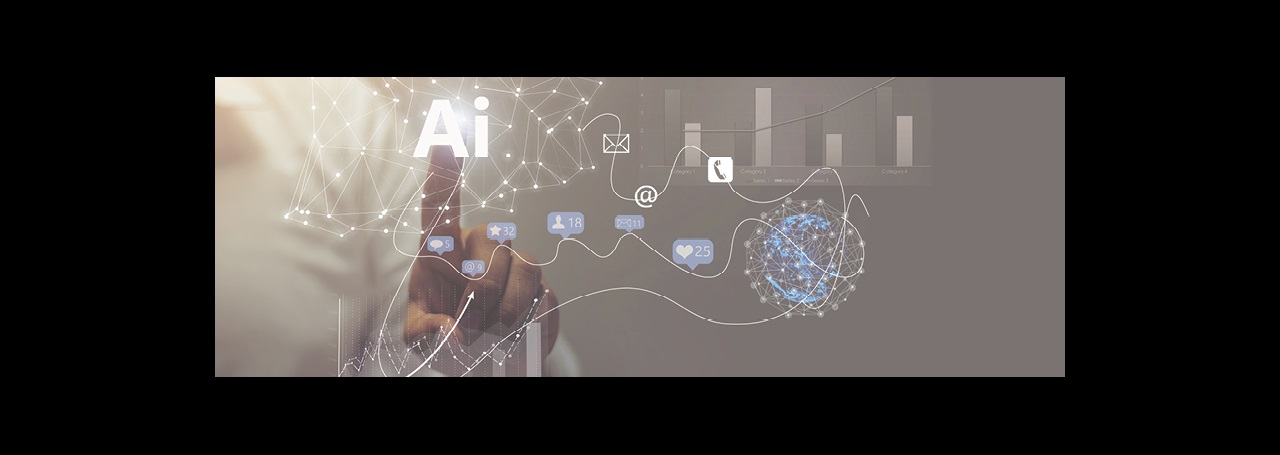
The operational challenges facing today’s hospitals and health systems are many. Yet, despite investing heavily in their EHR systems, many organizations still lack the tools to do more than manually extract and decipher data that simply highlights their issues rather than drives solutions.
Innovative artificial intelligence (AI) and machine learning (ML) technologies are helping healthcare organizations harness the data housed within EHRs to address capacity and other operational issues, better manage scarce human resources, and even prevent workforce burnout, all while improving patient experience.
How AI/ML Are Improving Operations: 3 Examples
Sophisticated AI and ML technologies are all around us. Airlines use them to predict how many passengers will fly from point A to point B and then price their fares accordingly. Delivery companies use them to load millions of packages onto trucks and airplanes efficiently and deliver them all over the globe. And as healthcare organizations begin to adopt AI/ML tools more frequently, they can use them to help address common operational headaches, including:
Tackling staffing shortages. It’s not uncommon for ORs across the country to underuse block time, yet they end up incurring overtime, according to Sanjeev Agrawal, president/COO, LeanTaaS, Santa Clara, Calif. “There are chunks of time when staffed ORs are not being used, and yet those same ORs are running late into the night and require staffing after hours,” he says. Predictive analytics can reveal openings to others who can fill those gaps.
AI-based solutions can help a hospital predict the number and kinds of cases that might be completed and when, which gives administrators the ability to reduce the ups and downs of clinicians’ schedules that can lead to burnout. Agrawal uses a popular COVID-era term to describe this solution. “By reorganizing the schedule, you flatten the curve and add a layer of predictability in clinicians’ workdays that allows them to see more patients, reduce wait times and become more efficient,” he says.
As a result, their workload flattens over the course of the day, which lessens the stress on clinicians. “There are times they don’t have a lot of cases, and there are times when they have to work overtime to accommodate caseload,” Agrawal says. “Scheduling appropriately is a workforce-satisfaction success.”
Addressing inpatient bed capacity. Artificial intelligence and machine learning tools improve predictability of length of stay and provide insights into which sets of patients are most likely to be discharged. “With AI, we can ‘bucket’ patients currently in beds with other people who medically resembled them when they were in the hospital and provide far better recommendations.” Better predictions about patients’ discharge dates can lead to better management of throughput across hospitals, as well.
Improving patient scheduling. Backlogs of patients who have delayed care during the pandemic and ongoing clinician shortages continue to trouble healthcare providers, with many patients waiting weeks or even months to schedule appointments with primary care providers and specialists. The problem, according to Agrawal, is not with office staff—it’s with the lack of predictions for the volume and mix of patients that are likely to show up to appointments. Schedulers typically make predictions based on certain traditional rules of thumb—book longer appointments in the morning and shorter ones in the afternoon, for example. This becomes a game of Tetris that can never truly be won due to the sheer number of possibilities involved, according to Agrawal. The solution? AI-based tools that use constraint-based optimization and can work through hundreds of possibilities to provide a template of sorts for medical offices to use to maximize the number of patients they can see in a day.
The Future Is Now
These technologies aren’t a fad, and they’re not a luxury, according to Agrawal. “The fundamentals of your business (access, cost structure, patient experience) are going to be driven by machine learning and AI embedded in sophisticated experiences delivered through the patient journey,” he says. “They are a necessity to become operationally efficient.”
Healthcare leaders who are planning to adopt these tools should create a budget for them that is distinct from their general IT budget, Agrawal recommends. It is like when the internet was first being adopted and everything was lumped into the “IT budget.” When organizations realized that every part of the business was going to be heavily influenced by the internet, they enabled each business unit to make their own tradeoffs between spending on digitization versus hiring more people to grow and scale, according to Agrawal. Similarly with ML/AI, giving business owners the ability to improve operations and grow their business will require some “client-funded projects” instead of everything being driven centrally, he says.
And don’t overlook the importance of supplier partnerships, which can provide important expertise to help hospitals or health systems get the most out of AI and ML technologies. The choice to adopt or not adopt these technologies might just come down to value. “If you think about just seeing one more patient per doctor per day or doing one more case per OR per week, that’s a lot of value,” Agrawal says. “The value at stake is billions of dollars in this industry.”
For more information, please contact Sanjeev Agrawal, president/COO, LeanTaaS, at sanjeev.a@leantaas.com.